Martin Danelljan
This site is not maintained!
Check my personal webpage: http://martin-danelljan.github.io
Biography
I received my Ph.D. degree from Linkoping University, Sweden in 2018. I am currently a postdoctoral researcher at ETH Zurich, Switzerland. My main research interests are online machine learning methods for visual tracking and video object segmentation, probabilistic models for point cloud registration, and machine learning with no or limited supervision.Research
ECO: Efficient Convolution Operators for Tracking, CVPR 2017In this work we tackle the key causes behind the problems of computational complexity and over-fitting in advanced DCF trackers.
Beyond Correlation Filters: Learning Continuous Convolution Operators for Visual Tracking, ECCV 2016 (oral)In this work we develop a theoretical framework for discriminatevly learning a convolution operator in the continuous spatial domain. Our formulation enables a natural integration of multi-resolution deep feature maps. In addition, our continuous formulation is capable of accurate sub-pixel localization of the target.
A Probabilistic Framework for Color-Based Point Set Registration, CVPR 2016In work we propose a probabilistic point set registration framework that exploits available color information associated with the points. Our method is based on a model of the joint distribution of 3D-point observations and their color information. We derive an EM algorithm for jointly estimating the model parameters and the relative transformations. The proposed model captures discriminative color information, while being computationally efficient.
Adaptive Decontamination of the Training Set: A Unified Formulation for Discriminative Visual Tracking, CVPR 2016In this work we propose a unified formulation for alleviating the problem of corrupted training samples in tracking-by-detection methods. This is achieved by minimizing a joint loss over both target appearance model and the training sample quality weights. Our approach is generic and can be integrated into any discriminative tracking framework.
Learning Spatially Regularized Correlation Filters for Visual Tracking, ICCV 2015In this work we propose Spatially Regularized Discriminative Correlation Filters (SRDCF) for tracking. This effectively mitigates the unwanted boundary effects, which limits the performance of standard correlation based trackers. The SRDCF tracker won the recent OpenCV Challenge and achieved the best result in the VOT-TIR2015 challenge.
Scale Estimation for Visual Tracking, TPAMI, BMVC 2014Here we investigate the problem of accurate and fast scale estimation for visual tracking. The proposed Discriminative Scale Space Tracker (DSST) won the Visual Object Tracking (VOT) 2014 challenge.
Coloring Visual Tracking, CVPR 2014 (oral)In this work, we investigated how to incorporate color information into visual tracking.
Publications
See my Google Scholar profile or my dblp page for a list of publications.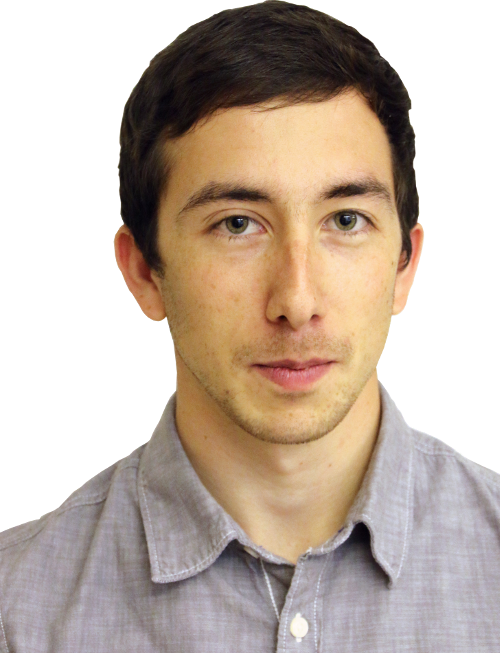
Martin Danelljan
Contact:
Postal address:
Computer Vision Laboratory
Department of Electrical Engineering
SE-581 83 Linköping, Sweden
Phone:
+46(0)13-28 18 49
Visit me at:
Building B, Campus Valla
Room 2A:470
- first floor, corridor A,
- between entrances B27 and B29
< >
My pages:
Page responsible: Martin Danelljan
Last updated: 2019-04-15