Archived news
- Oct 29, 2017: We are proud to announce the Board Game Finder, a recommender system for board games powered by machine learning.
- May 31, 2017: We have published Variational Sequential Monte Carlo, a new variational approximating family that combines sequential Monte Carlo with variational Bayes.
- Apr 20, 2017: We were awarded the AISTATS 2017 Best Paper Award (link) for the paper Reparameterization Gradients through Acceptance-Rejection Sampling Algorithms.
- Aug 12, 2016: I have been awarded a Fulbright grant and will spend the academic year 2016/2017 as a visiting student researcher in Prof. David M. Blei's research lab at Columbia University in the City of New York.
- Jun 17, 2016: Next week I will be attending ICML in New York! We will be presenting our new algorithm Interacting Particle Markov Chain Monte Carlo, especially useful for probabilistic programming. The method is implemented on the development branch of the probabilistic programming system Anglican.
-
Apr 25, 2016: The new particle MCMC method we have developed, the Interacting Particle Markov Chain Monte Carlo, which efficiently makes use of distributed and multi-core computing architectures has been accepted to ICML 2016. You can find a pre-print on arXiv.
- Dec 1, 2015: Next week I will be attending NIPS in Montreal, Canada. I will be presenting two posters at the "Black Box Inference and Learning" and "Scalable Monte Carlo Methods" workshops. One is about Hamiltonian dynamics and its use in importance sampling-based algorithms, and the second one is work towards automating SMC inference for probabilistic programs and graphical models. They can both be found here.
- Oct 12, 2015: Next week I will be visiting Frank Wood's group at the University of Oxford. I will also be giving a talk on Monte Carlo methods (focus on importance sampling-based versions) and proper weighting.
-
Jun 1, 2015: Our tutorial paper Sequential Monte Carlo Methods for System Identification was accepted to SYSID2015, pre-print available on arXiv. In this paper we describe different approaches (both Bayesian and frequentist) to estimate parameters in general state space models, also know as hidden Markov models. The SMC approach is motivated by the intractability of general non-Gaussian (non-discrete) state space models.
Apr 27, 2015: Our paper entitled Nested Sequential Monte Carlo Methods has just been accepted to the International Conference on Machine Learning! In this paper we propose a method to efficiently approximate the locally optimal (fully adapted) particle filter using nested Monte Carlo methods. We show that this new method can handle filtering problems of much higher dimension than the standard particle filter. A pre-print is available here or on arXiv. The picture above displays marginal posterior approximation to a 600-dimensional filtering problem.- Mar 25, 2015: Our tutorial paper Sequential Monte Carlo Methods for System Identification is now available as a pre-print on arXiv. In this paper we describe different approaches (both Bayesian and frequentist) to estimate parameters in general state space models, also know as hidden Markov models. The SMC approach is motivated by the intractability of general non-Gaussian (non-discrete) state space models.
- Feb 9, 2015: We have just published a pre-print entitled Nested Sequential Monte Carlo Methods available here or on arXiv. In this paper we generalise the SMC framework by only requiring approximate, properly weighted, samples from the proposal distribution, while still resulting in a correct SMC algorithm. We show that this new method can handle filtering problems of much higher dimension than the standard particle filter.
- Nov 26, 2014: In December, I will attend NIPS (homepage) in Montreal, Canada. I will present a poster on our paper Sequential Monte Carlo for Graphical Models. The paper and link to code used to produce the results can be found here.
- Oct 22, 2014: In November, I will attend the Information Theory Workshop (homepage) in Hobart, Australia. During my stay in Australia I will visit with Pierre del Moral at UNSW, Sydney and Brett Ninness at University of Newcastle. I will also give talks on our work on probabilistic graphical models.
- Sep 16, 2014: I will attend Bayesian Young Statisticians Meeting (homepage) in Vienna this week and European Research Network on System Identification workshop (homepage) in Ostend next week. On both occasions I will present posters on our recent work on sequential Monte Carlo methods for probabilistic graphical models. The posters can be found here.
- Sep 9, 2014: More great news, our paper on sequential Monte Carlo (SMC) methods for probabilistic graphical models (PGM) was accepted to NIPS 2014! We propose a novel way to use SMC within the PGM framework to do inference, partition function (normalisation constant) estimation and hint at possible extensions to Bayesian learning of parameters. A pre-print can be found here [C2].
- Sep 1, 2014: I recently won the best poster award at the Summer school on deep learning for image analysis for our work on sequential Monte Carlo methods for (Probabilistic) Graphical Models. The poster [P3] is available here and related publications ([C2] and [C3]) are available here.
- Jul 27, 2014: Our paper Capacity estimation of two-dimensional channels using Sequential Monte Carlo has been accepted for publication at the 2014 IEEE Information Theory Workshop held in Hobart, Australia in November. Preprint available here: [arXiv]
- Jun 23, 2014: We present a new sequential Monte Carlo algorithm based on the divide-and-conquer methodology. The basic idea is to start with independent particle populations, which are then combined and merged depending on the structure of the graphical model. Promising results are presented for a MRF and hierarchical model. [arXiv]
- Jun 10, 2014: Major update of our framework for using SMC methods for inference and learning in graphical models. Thorough experimental section profiling our methods with state-of-the-art on interesting problems, both real and simulated data. [arXiv]
- May 2, 2014: We propose to use a fully adapted SMC algorithm to estimate channel capacity in 2-D channel models. We show how calculating the resampling weights and simulating from the optimal proposal can be performed efficiently using Forward Filtering/Backward Sampling. The results on the 2-D one-infinity run-length limited constrained channel show improvement of more than an order of magnitude MSE compared to state-of-the-art. [arXiv]
- Feb 3, 2014: We propose a new way of using SMC methods for inference and learning in graphical models. The results are promising and the method applies to graphs of any topology as well as both continuous and/or discrete valued variables. [arXiv]
For more information about my work please see the publications, presentations and source code pages.
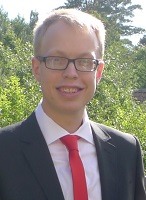
PhD Student in Automatic Control
(Swedish: Doktorand i reglerteknik)
- Phone:
- +46 13 281087
- E-mail:
- christian.a.naesseth_at_liu.se
- Address:
- Dept. of Electrical Engineering
- Linköping University
- SE-581 83 Linköping
- Sweden
- Visiting Address:
- Campus Valla
- Building B
- Room 2A:522 (in the A corridor on the ground floor between entrance 25 and 27)
Informationsansvarig: Christian Andersson Naesseth
Senast uppdaterad: 2018-09-11